What are the big data ubiquity business practice guides?
In the era we are in, data is everywhere. According to IBM estimates, humans generate about 25,000 ZB of data per day, which means that 90% of the world's data is generated in the past two years. Gartner's analysis shows that 85 percent of Fortune 500 companies in 2015 are unable to leverage big data to gain a competitive advantage.
Let's take a few examples. Financial institutions use the credit scoring model to understand the credits of their customers on each credit product (mortgage, credit card, installment loan) in the next 12 months. They use this credit score to make bad debt provision, calculate the amount of capital required by Basel II/III, or develop a marketing plan (such as adjusting credit card limits based on credit scores).
Telecom operators use the recent call behavior data to establish a churn model, estimating the likelihood that customers will lose in the next one to three months. Operators will develop marketing campaigns based on model scores to avoid valuable customer churn. Facebook and Twitter use social media analytics to conduct content analysis and emotional semantic analysis to better understand brand awareness and further tune product service design.
Online retailers such as Amazon and Netflix continually analyze customer buying behavior to determine product bundling strategies and use the recommendation system to purchase recommended products for customers next time. Credit card companies use fraud detection models to detect fraudulent payments and whether credit card theft has occurred. The government uses data analysis technology to predict tax evasion behavior, optimize public budget allocation, analyze traffic data to improve public transportation efficiency, and analyze and predict terrorist attacks to ensure national security.
Data for the value <br> <br> data is the foundation of any successful model. When starting an analysis project, it is necessary to detail all the data available for analysis in the enterprise. The principle here is that the more data the better! Because many analytical models automatically determine which data is important for the current analysis and which data can be excluded from the next analysis.
Our research continues to confirm the view that the best way to improve your analytical model is to invest in your data! This can be done by raising the two dimensions of quantity and quality. For the former, a key point is how to integrate structured data (such as relational databases) and unstructured data (such as text) to provide a comprehensive perspective for customer behavior analysis. Another key point is the integration of online data and offline data. Many companies are troubled by this problem.
In addition, companies can go beyond their internal boundaries and consider purchasing external data from external data providers to compensate for the lack of internal data. A large number of studies have shown that the use of external data is very useful for comparing and refining analytical models. Although data is often massive, data quality is often a pain point. The principle of GIGO (garbage in garbage out) is very useful here, and rotten data will only generate rotten models.
It sounds obvious, but in practice data quality often becomes the “Achilles' heel†for many analytical projects. Data quality can be broken down into many dimensions: accuracy, integrity, recency, consistency, and more. In big data analysis, companies must specifically develop data quality management solutions, set up data auditors, data stewards or data quality managers to continuously monitor data quality.
Data analysis should start with business issues, not with specific technical solutions. But this has the meaning of "chicken eggs, eggs and chickens." To solve business problems and identify business opportunities, you need to understand the potential technical solutions. Take social media analysis as an example. Only after first understanding the analysis technology can the company begin to think about how to use them to study online brand awareness and monitor trends. In order to bridge the gap between analytics technology and business, continuous training and learning are key, enabling companies to remain at the forefront of analytics technology and maintain a competitive edge. At this point, the academic community should be deeply reviewed because many of the existing master's programs in big data analysis (or data science) cannot meet the above requirements.
Another key point in turning data into insight and enhancing value is the validation of the analytical model. Analytical models require appropriate mechanisms and tools for review and validation, and more and more companies are splitting the analysis team into two teams, model development and model validation. Good corporate governance creates a firewall between the two teams, allowing models developed by the previous team to be objectively and independently evaluated by the latter team.
Companies can even consider model validation by external partners. By establishing an analytical basic information facility, the company is able to continuously evaluate and validate models based on existing conditions, improve the performance of analytical models, and capture more target customers.
Data analysis is often not a one-time thing. In fact, when the analytical model is put into use, it is out of date! Analytical models are always behind reality, all we can do is keep this lag as small as possible. The data used to analyze the model is collected at a specific point in time and under specific internal and external environmental conditions.
This particular environment is not static, but changes with internal factors (such as new strategies, changing customer behavior) and external factors (new macroeconomic environment and laws and regulations). For example, in fraud detection analysis, fraudsters always try to evade detection of models to defraud more money. Another example is that the credit scoring model relies heavily on current macroeconomic conditions (recovery or recession). Therefore, in order for the analytical model to be successful in creating value, it is necessary to monitor the internal and external environment and adjust or reconstruct the model in time.
Construction of underlying <br> <br> order to establish an analytical environment, companies need to be selected on the hardware and software technology adopted.
On the hardware side, specialized infrastructure (such as Hadoop and associated software stacks) is needed to clean, consolidate, store, and manage data. To reduce expenses, companies may choose cloud storage and use big data as a service. When the company conducts data transmission and exchange with the outside, appropriate precautions should be taken to ensure data privacy.
On the software side, many vendors offer commercial solutions for big data analytics. There are still a lot of open source analysis software (R, weka, Rapidminer) in the market. Although these open source software solutions have become very popular, they are not mature enough to handle the characteristics of mass and diversity. Big Data.
Big data analysis has become a part of the DNA of more and more companies, but government, finance, and medicine have their own development footprints. Data, business, and regulation are specific and need to be set differently for different genetic components. . So what companies need is software that provides a comprehensive, comprehensive vertical business solution, rather than a cross-industry, horizontally-available software.
Given the complexity of establishing a data analytics architecture environment, companies may consider outsourcing. However, the company's internal data and the information it contains are the company's most valuable strategic assets, so it is not appropriate for third parties to have full access to data. Instead, companies should build internal analytical centers and develop analytical skills for company personnel to serve the company's broad analytical needs, a problem that companies should consider in their management.
Similarly, the company's board of directors and senior management should be involved in the analysis of the environment. Many companies are also trying to set up positions such as the Chief Analyst (CAO), which is responsible for establishing an enterprise-wide analytical environment and infrastructure, and managing the development, review, and deployment of analytical models for each business unit.
Finally, we are now seeing more and more small and medium-sized businesses starting to use big data analytics. These companies typically have limited budgets, so they prefer existing off-the-shelf software solutions that can be used directly for data analysis. For example, use online analysis tools to study the use of websites, optimize website settings, improve website rankings in search engines, and purchase paid engine marketing solutions.
Summary and Outlook <br> <br> place at the end of the article, we would like to reiterate enterprise big data analysis several problems must pay attention again:
From the perspective of company management:
(1) The company should pay attention to both data volume and data quality;
(2) Continuous learning and training can bridge the gap between new analytical techniques and new business opportunities;
(3) The analytical development team should include an independent model development team and a model validation team;
(4) Analysis is not only model development and verification, but also model monitoring and backtesting.
From a technical perspective, the company should:
(1) Consider using cloud services for big data analysis;
(2) should pay attention to vertical industry solutions, carefully choose open source software;
(3) Be cautious when dealing with outsourcing analysis. It is best to establish an analysis environment within the enterprise and place it under high-level management.
What are the big data ubiquity business practice guides?
Data is everywhere
By 2020, there will be millions of data-related jobs in the world. These vast amounts of data hold valuable wealth, and companies can use the most advanced analytics to use them to better understand customer behavior, identify business opportunities, and develop operational strategies.Let's take a few examples. Financial institutions use the credit scoring model to understand the credits of their customers on each credit product (mortgage, credit card, installment loan) in the next 12 months. They use this credit score to make bad debt provision, calculate the amount of capital required by Basel II/III, or develop a marketing plan (such as adjusting credit card limits based on credit scores).
Telecom operators use the recent call behavior data to establish a churn model, estimating the likelihood that customers will lose in the next one to three months. Operators will develop marketing campaigns based on model scores to avoid valuable customer churn. Facebook and Twitter use social media analytics to conduct content analysis and emotional semantic analysis to better understand brand awareness and further tune product service design.
Online retailers such as Amazon and Netflix continually analyze customer buying behavior to determine product bundling strategies and use the recommendation system to purchase recommended products for customers next time. Credit card companies use fraud detection models to detect fraudulent payments and whether credit card theft has occurred. The government uses data analysis technology to predict tax evasion behavior, optimize public budget allocation, analyze traffic data to improve public transportation efficiency, and analyze and predict terrorist attacks to ensure national security.
Data for the value <br> <br> data is the foundation of any successful model. When starting an analysis project, it is necessary to detail all the data available for analysis in the enterprise. The principle here is that the more data the better! Because many analytical models automatically determine which data is important for the current analysis and which data can be excluded from the next analysis.
Our research continues to confirm the view that the best way to improve your analytical model is to invest in your data! This can be done by raising the two dimensions of quantity and quality. For the former, a key point is how to integrate structured data (such as relational databases) and unstructured data (such as text) to provide a comprehensive perspective for customer behavior analysis. Another key point is the integration of online data and offline data. Many companies are troubled by this problem.
In addition, companies can go beyond their internal boundaries and consider purchasing external data from external data providers to compensate for the lack of internal data. A large number of studies have shown that the use of external data is very useful for comparing and refining analytical models. Although data is often massive, data quality is often a pain point. The principle of GIGO (garbage in garbage out) is very useful here, and rotten data will only generate rotten models.
It sounds obvious, but in practice data quality often becomes the “Achilles' heel†for many analytical projects. Data quality can be broken down into many dimensions: accuracy, integrity, recency, consistency, and more. In big data analysis, companies must specifically develop data quality management solutions, set up data auditors, data stewards or data quality managers to continuously monitor data quality.
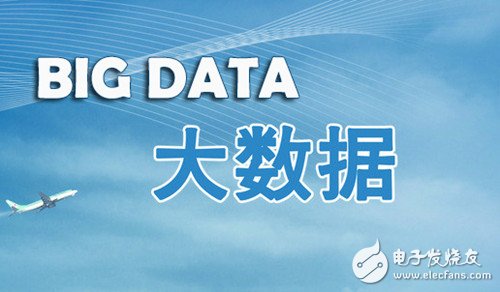
Another key point in turning data into insight and enhancing value is the validation of the analytical model. Analytical models require appropriate mechanisms and tools for review and validation, and more and more companies are splitting the analysis team into two teams, model development and model validation. Good corporate governance creates a firewall between the two teams, allowing models developed by the previous team to be objectively and independently evaluated by the latter team.
Companies can even consider model validation by external partners. By establishing an analytical basic information facility, the company is able to continuously evaluate and validate models based on existing conditions, improve the performance of analytical models, and capture more target customers.
Data analysis is often not a one-time thing. In fact, when the analytical model is put into use, it is out of date! Analytical models are always behind reality, all we can do is keep this lag as small as possible. The data used to analyze the model is collected at a specific point in time and under specific internal and external environmental conditions.
This particular environment is not static, but changes with internal factors (such as new strategies, changing customer behavior) and external factors (new macroeconomic environment and laws and regulations). For example, in fraud detection analysis, fraudsters always try to evade detection of models to defraud more money. Another example is that the credit scoring model relies heavily on current macroeconomic conditions (recovery or recession). Therefore, in order for the analytical model to be successful in creating value, it is necessary to monitor the internal and external environment and adjust or reconstruct the model in time.
Construction of underlying <br> <br> order to establish an analytical environment, companies need to be selected on the hardware and software technology adopted.
On the hardware side, specialized infrastructure (such as Hadoop and associated software stacks) is needed to clean, consolidate, store, and manage data. To reduce expenses, companies may choose cloud storage and use big data as a service. When the company conducts data transmission and exchange with the outside, appropriate precautions should be taken to ensure data privacy.
On the software side, many vendors offer commercial solutions for big data analytics. There are still a lot of open source analysis software (R, weka, Rapidminer) in the market. Although these open source software solutions have become very popular, they are not mature enough to handle the characteristics of mass and diversity. Big Data.
Big data analysis has become a part of the DNA of more and more companies, but government, finance, and medicine have their own development footprints. Data, business, and regulation are specific and need to be set differently for different genetic components. . So what companies need is software that provides a comprehensive, comprehensive vertical business solution, rather than a cross-industry, horizontally-available software.
Given the complexity of establishing a data analytics architecture environment, companies may consider outsourcing. However, the company's internal data and the information it contains are the company's most valuable strategic assets, so it is not appropriate for third parties to have full access to data. Instead, companies should build internal analytical centers and develop analytical skills for company personnel to serve the company's broad analytical needs, a problem that companies should consider in their management.
Similarly, the company's board of directors and senior management should be involved in the analysis of the environment. Many companies are also trying to set up positions such as the Chief Analyst (CAO), which is responsible for establishing an enterprise-wide analytical environment and infrastructure, and managing the development, review, and deployment of analytical models for each business unit.
Finally, we are now seeing more and more small and medium-sized businesses starting to use big data analytics. These companies typically have limited budgets, so they prefer existing off-the-shelf software solutions that can be used directly for data analysis. For example, use online analysis tools to study the use of websites, optimize website settings, improve website rankings in search engines, and purchase paid engine marketing solutions.
Summary and Outlook <br> <br> place at the end of the article, we would like to reiterate enterprise big data analysis several problems must pay attention again:
From the perspective of company management:
(1) The company should pay attention to both data volume and data quality;
(2) Continuous learning and training can bridge the gap between new analytical techniques and new business opportunities;
(3) The analytical development team should include an independent model development team and a model validation team;
(4) Analysis is not only model development and verification, but also model monitoring and backtesting.
From a technical perspective, the company should:
(1) Consider using cloud services for big data analysis;
(2) should pay attention to vertical industry solutions, carefully choose open source software;
(3) Be cautious when dealing with outsourcing analysis. It is best to establish an analysis environment within the enterprise and place it under high-level management.
A Home Projector that supports WiFi connection, usually an Android system, can connect to the same WiFi to realize screen sharing. In addition to multimedia functions, you can also watch videos, download software and play games online. Mainly focusing on high-quality goods, the projection screen is clear and true, suitable for home, office, teaching, and entertainment
wifi mini home projector,wifi protable home projector,bluetooth mini projector,HD mini projector
Shenzhen Happybate Trading Co.,LTD , https://www.happybateprojector.com